
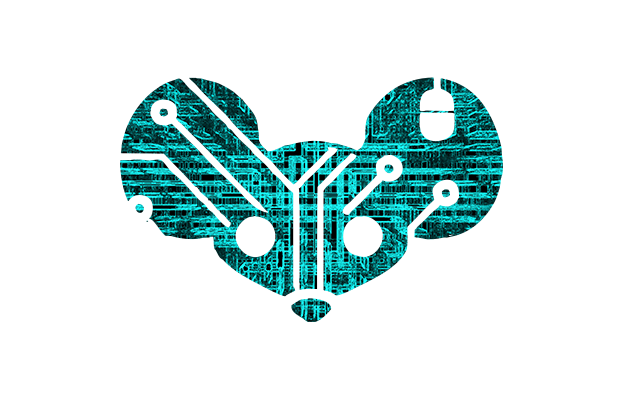
Human drivers are well known for never ever harassing women.
Human drivers are well known for never ever harassing women.
They offer a sound option right below.
I have been without seeing tv news for many years now. I get my news in written format.
Everytime I stumble onto some video I don’t understand how people don’t understand all the million manipulations that are present in every single second of video.
Text is also manipulated but it is way easier to read over the manipulations, to fact check and to collect different sources of information.
My poor european mind cannot understand the industrial bottle of 1000 ibuprofen tablets for a household.
Here they sell you packs of 20 tablets or something like that.
The thing is that we need a better donation system, for people to trust it.
An auditable system, and goal oriented.
I’m tired of donating to something and seeing that instead of the good project I wanted my money went to some crazy side project or to some over the top salaries for high corporate.
We need some kind of trustable platform that audits where donation money goes, and enforces binding of the donated money for the purpose it was donated for.
I got really burned with the whole wikiMedia thing. And since them I’m very cautious to who I donate to.
There is only one linux kernel.
You really shouldn’t. Cops are never on your side.
I mean never is a strong word here. People are saved by cops every day. At least in my country. Just looking today news: women was arrested after being violent towards doctors in La Palma. Pretty sure doctors though cops were on their side.
You have to be sure to defend your citizen rights and prosecuted rights (if it even become to that). But that does not conflict to call the cops if you need to and if you are being victim of a crime they’ll most likely help you. Once again, that’s how it is where I live.
Remember to contact your political representative and express your concerns on any public organization account having an account on twitter. Also contact any journali of a media you use to read/watch and express the same concern.
Once politics and journalists get out of twitter is game over.
If you read my answers you’ll see that I said they are not llm. They are language models powered by smaller datasets and with smaller neural networks.
I picked a tax agency in particular because I know first hand that tax agencies (I would surprise me that UK didn’t use it) do use language models with neural networks, notice that again I’m not saying generative llm, to parse the question and select a proper answer. Not the keyword method you think they use.
I would have provided the first hand example I know but it is spanish and people may not be able to effectively understand it. But I do know that tax agencies usually use very similar tools one country from another. So probably UK does use it. If you want to test the spanish one here it is. And sources on what type of AI is used.
https://sede.agenciatributaria.gob.es/Sede/ayuda/herramientas-asistencia-virtual.html
Again, because it seems that I need to repeat this so people can properly train on the info I’m writing, not LLM, not GPT, not a large general use language model. As for that amount of parameters cutting not confident answers would cut most answers, probably. At least with nowadays state of technology, things keep improving each year.
Edit: found some english source on the matter https://www.investinspain.org/en/news/2024/ibm
The chatbot it is still only in spanish and co-official languages still.
Like taking your phone and go through your WhatsApp messages?
If that’s a concern you could set up a password to access any sensible app or chat within that app.
I think that is a more sensible approach. As if you are targeted by any reason an undercover cop could get a hold on your unlocked phone by many different ways.
More or less that. There’s a point during the path that the input is taking on the language model were the induced randomness can significantly affect the output or not. If all the weights are pointing to the same end node, because the “confidence” is high, the no matter the random seed, the output will be the same. When the seed greatly affect the final result is because the weights don’t point with that confidence to an unique end node, so the small randomness introduced at the beginning (the seed to say so) greatly change the result. It is here were you are most likely to get an hallucination.
To put again in terms of the much more easier to view earlier neural networks. When you didn’t trail the model enough mario just made random movements without doing attempts to complete the level. Because the weights of the neurons could not reliably take the input and transform into an useful output. It os something that could be solved in smaller models. For larger models gets incredibly complicated because the massive amount of data. The complexity of the data. And the complexity of a proper training. But it’s not something imposible or that could not get rid of. The same you can get Mario to finally complete all levels every time without issues, you can get a non hallucinanting chat bot, it just takes more technology improvements.
I suppose it could be said that the nature of language is chaotic like weather and not deterministic like a Mario level, and thus it would be actually “impossible” to get large results, like it’s impossible to get precise weather a month in advance. But I’m not sure there would be enough evidence to support that, as hallucinations are not just across the board, they just tend to happen on matters that had little training data. Matters with plenty of training data do not hallucinate even in today models.
I searched slm online and found out that small models you said. I wasn’t refering to those. Those are just small large language models IMO if that makes any sense. A proper slm should also have a small purpose, cannot be general chat. I mostly refer to the current chatbots that point you to predefined answers, or summarizing ones. Nothing that could really elaborate a wrote answer word by word.
Currently and to my knowledge. There isn’t any general language model that can just write up answers and that is good enough to not hallucinate. But certainly we are getting closer each year.
Edit: I’ve been looking for an example, here https://www.tax.service.gov.uk/ask-hmrc/chat/self-assessment These kind of chatbots, they know when their answer is not precise and default to a polite “ask again” answer instead of just tell you the first “hallucination” that came to them. They are powered by similar AI technology but it’s not a general use and cannot write word by word. But it “knows” when te answer is precise or not.
Of course. But if a police officer were to remove your phone by force, first it would be illegal without a warrant so it would almost made you a favour as all evidence in your phone would be invalid in court.
Then if they just want to remove by force, with or without warrant, they can just take it from your pocket. Even locked if they want the info in your phone they are probably getting it. They would have access to some of the best forensics teams and equipment.
Following the same logic, should we never have an unlocked phone near a police officer? I don’t know about that.
And if you are just that paranoid I would probably be easy to just have a second profile on your phone just for the ID. And you are the same as if having the phone locked as password is needed for changing profiles.
What do you think is “weight”?
Is, simplifying, the amounts of data that says “The capital of France is Paris” it doesn’t need to understand anything. It just has to stop the process if the statistics don’t not provide enough to continue with confidence. If the data is all over the place and you have several “The capital of France is Berlin/Madrid/Milan”, it’s measurable compared to all data saying it is Paris. Not need for any kind of “understanding” of the meaning of the individual words, just measuring confidence on what next word should be.
Back a couple of years when we played with small neural networks playing mario and you could see the internal process in real time, as there where not that many layers. It was evident how the process and the levels of confidence changed depending on how deep the training was. Here it is just orders of magnitude above. But nothing imposible to overcome as some people pretend to sell.
Alternative ways of measure confidence is just run the same question several times and check if answers are equivalent.
PhD is PhD in scaremongering about technology, so it’s not an authority on anything here.
IDK what did you do, but slm don’t really hallucinate that much, if at all. Specially if they are trained with good datasets.
As I said the solution is not in my hand, as it involves improving the efficiency or the amount of data. Efficiency has issues as current techniques seems to be unable to improve efficiency over a certain level. And amount of data is, obviously, costly.
The Hidrogen from water thing is simply wrong. If that is supposed to mean that hallucinations are just part of a generative LLM technology that cannot be solved.
They are not inherent of the technology. They are a product of lack of control over the stadistical output. Prioritizing any answer before no answer.
As with any statistics you have a confidence on how true something is based on your data. It’s just a matter of putting the threshold higher or lower.
If you ask an easy question “What is the capital of France?” You wont ever get an hallucination. Because all models will have that answer provided with very high confidence. You just have to make so if that level of confidence is not reached it just default to a “I don’t know answer”. But, once again, this will make the chatbots seem very dumb as they will answer with lots of “I don’t know”.
The problem here is the amount of data and the efficiency of the model. In order to get an usable general purpose model with a confidence threshold high enough to not hallucinate, by todays efficiency with the models it would need to be an humongous model, too big and with too much training data even for big tech. So we can go that big, we can try to improve efficiency (which is being proven very hard for general models) or we do both. Time will tell, but I’m quite confident that we will reach a general use model without hallucinations sooner or later.
Is article mixing things or am I missing something?
You don’t need to hand over your phone to present a digital ID. At least in my country the digital ID just creates a qr that the cop can scan to verify. There is no reason to hand over anything on the whole process.
It actually can be fixed. There is an accuracy to answers. Like how confident the statistical model is on the answer. That’s why some questions get consistent answers while others don’t.
The fix is not that hard, it’s a matter of reputation on having the chatbot answer “I don’t know” when the confidence on an answer isn’t high enough. It’s pretty similar on what the chatbot does when you ask them to make you a bomb, just highjacks the answer calculated by the model and says a predefined answer instead.
But it makes the AI look bad. So most public available models just answer anything even if they are not confident about it. Also your reaction to the incorrect answer is used to train the model better so it’s not even efficient for they to stop the hallucinations on their product. But it can be done.
Models used by companies usually have a higher confidence threshold and answer “I don’t know” if they don’t have enough statistical proof on a particular answer.
Spanish people stopped learning greek after smartphones where invented.
We used to read shampoo bottles that where usually shipped in Spain with labels in four languages: Spanish, Italian, Portuguese and Greek. So it was always funny to try to see how things were written in greek while pooping.
I does not conflict with people being paid for doing something. If I’m a carpenter and I’m hired and paid to make a bench. No one is stealing me if, once the bench is done, I don’t have a said in who sits on that bench. Or if I don’t get paid every time a person sits on that bench.
Like any other job you should be paid for doing work. Not for owning a property.
I just don’t believe in copyright, IP patents or having fences on human culture.
So even if they straight up put Pikachu in their game I think they have the moral right to do so. If they can make a good game with Pikachu in it, who is Nintendo to private humanity from that piece of culture?
My statement is about morality. What’s legal or not is another matter.
They do not state the methodology used (paywalled is the same as not existent to me).
So probably it’s just not true.